Rational Drowning

The biggest mistake biotech teams make when it comes to improving their data practices is waiting for the right time to start. There’s always a higher priority problem or a reason to wait. But the longer you push it off, the harder it gets. Until one day, you look up and realize all your most important data is spread across laptop hard drives and shared folders with arbitrary names created by people who left the company months or years ago.
Almost every biotech is guilty of this. And they all have good reasons they tell themselves.
Early in the life cycle of a biotech, it feels like you’re iterating too fast to impose any kind of consistency. Experiment protocols are changing daily. You may not even have settled on which assays to use long-term. You can’t standardize data practices if the data itself isn’t standardized.
As things start to get more consistent and repeatable, you just don’t have the time to define processes and conventions. There’s always a tight deadline coming up or a fire to put out. So you wait for things to stabilize. You wait for when it’s time to scale.
Eventually, things really start to settle down and you start to think maybe you’re fine as is: Everyone seems to know how to find the data they need. Sometimes they may have to ask around a little, but it always works out. Plus, things have gotten complex enough that implementing a consistent system seems more disruptive than helpful. You’d have to convince multiple teams to change how they work, then sift through all the old data. Why put all that effort into fixing something that isn’t broken?
And then, one day you discover it was actually broken all along.
One company we talked to lost the data from their first large-scale screening run for over three months when the one person who knew where it was left the company. They only found it because they happened to re-hire a contractor who was involved in the original project.
Another spent six months dealing with consistent experiment failures from a new automated system that had cost hundreds of thousands of dollars to build. It wasn’t until they invested in organizing and analyzing the data that they discovered the culprit: The sun heating the room caused every experiment that ran after 11am to fail.
Then there are more examples than we can count of companies spending a large chunk of their budget on an experiment, only to discover that another team generated the exact same data a year earlier.
And these are only the ones that teams were willing to talk about. We’ll never know how many ill-fated biotech startups selected the wrong lead because data was copied into the wrong cell, or the wrong spreadsheet was used for an analysis.
Good data practices are an insurance policy against these kinds of mishaps. Like insurance, their day-to-day cost doesn’t always seem worth it day to day. But they more than make up for that cost in the money you don’t spend re-running experiments and going down avoidable dead ends.
So when it comes to improving your team’s data practices, it isn’t a question when to start. It’s a question of how.
Each stage in a biotech startup’s evolution requires a different set of data management practices. Early on, they need to be flexible enough to handle exploration and iteration. Later on, they need to support and enforce a growing level of consistency. And at every stage, they need to minimize the extra work for scientists. Ideally, they’ll actually make less work.
Sphinx was designed with exactly this balance of flexibility and automation in mind. Sphinx’s AI agent, Metis, helps scientists define data transformations to quickly get new and evolving datasets ready for analysis, while creating a detailed record of every analysis and every dataset. Then, when things start to settle down, Sphinx’s analysis templates allow scientists to quickly and flexibly run more consistent analysis, freeing up time to dig deeper.
When it comes down to it, biotech startups don’t adopt better data management practices because they don’t think it’s practically possible. Sphinx makes it more than possible - it makes it so easy, you won’t believe it
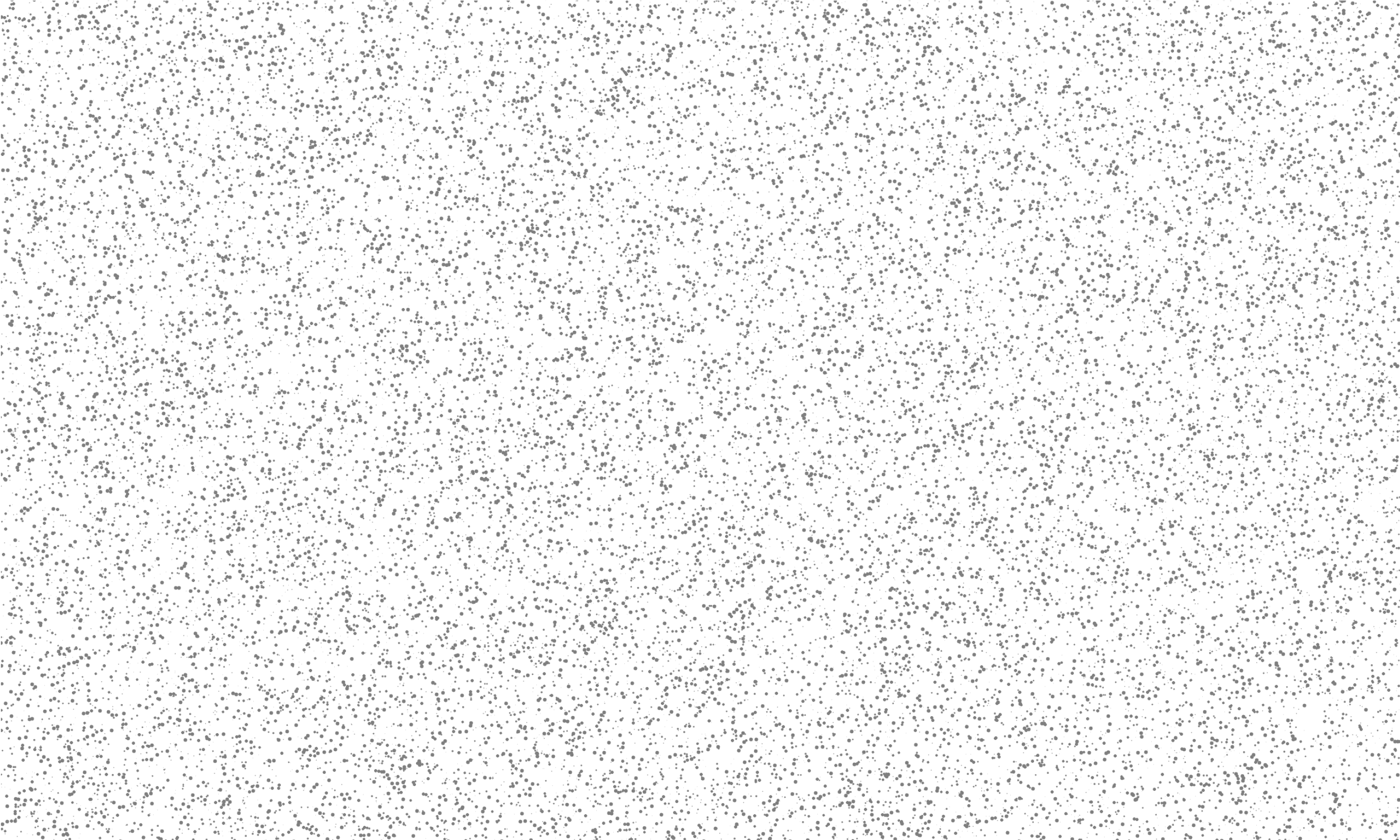
Additional Resources
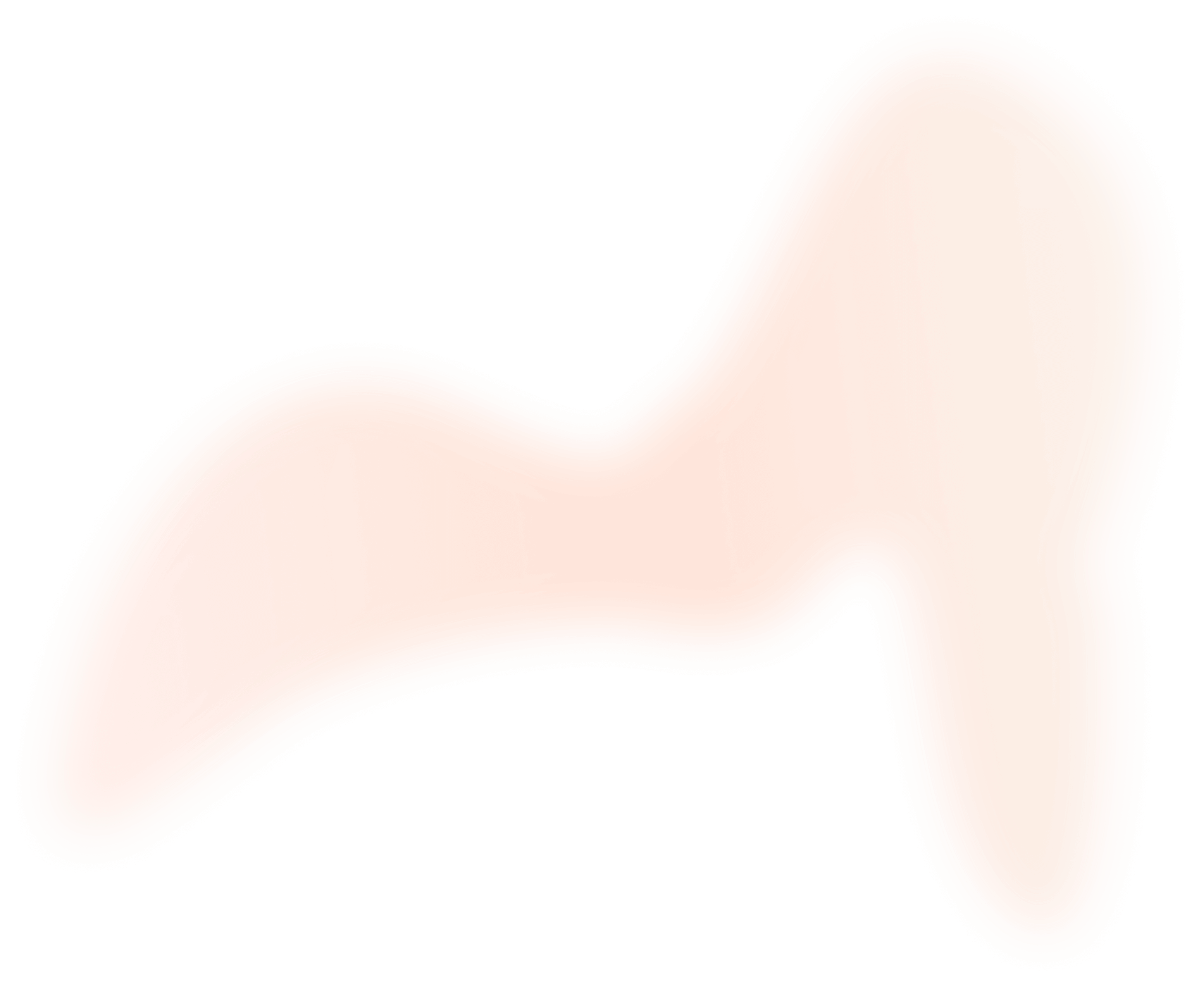