When computational biology becomes just biology
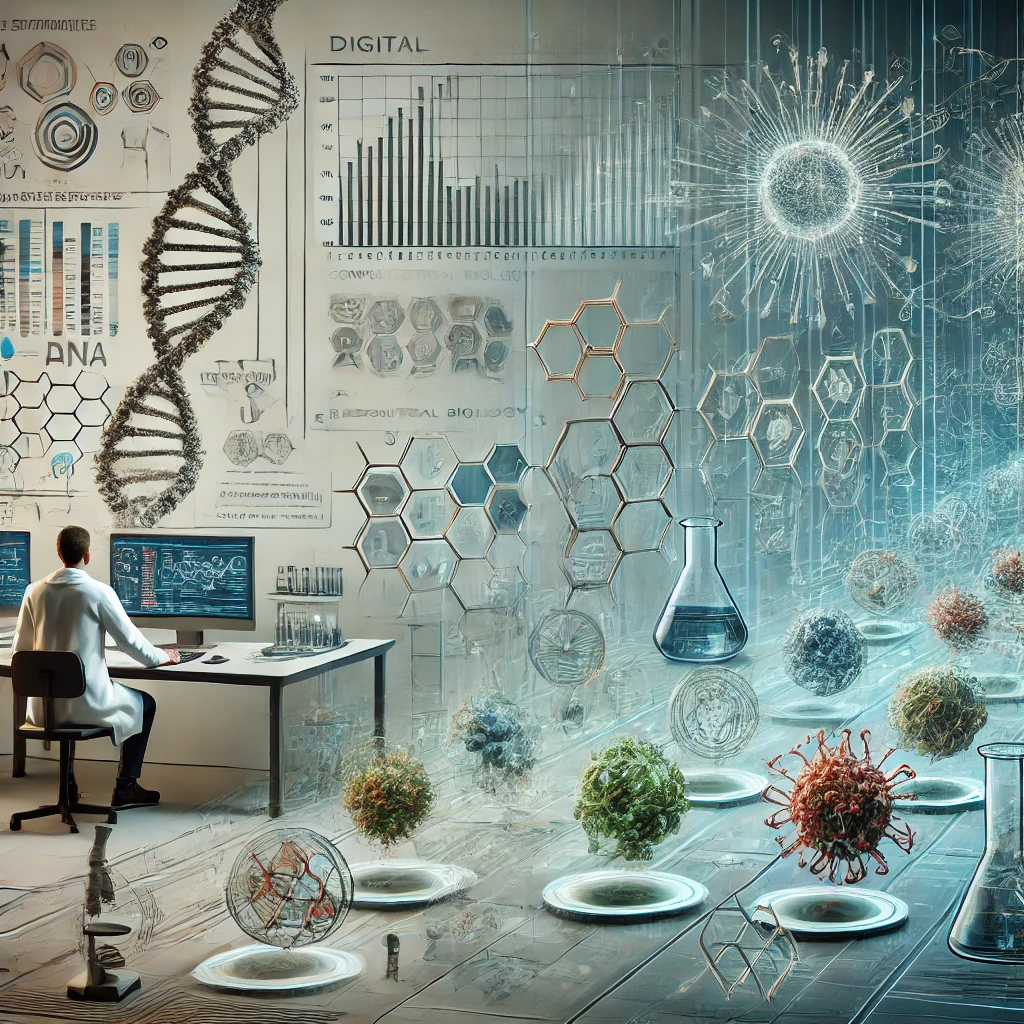
The biggest shift that separates the current state of biology from just a few decades ago isn’t new types of data, new algorithms or new models. It’s that a large part of the traditional role of biologist has been split off into a new role called a computational biologist. This split was necessary because the new role requires expertise with data and algorithms that classically trained biologists just don’t have. But it also adds a host of complexity and difficulty. Luckily, there’s reason to believe that this split will soon be a thing of the past.
The problems that arise from splitting the traditional role of a biologist aren’t hard to find: Another person or another team that needs to be kept in the loop multiplies (not just adds to) the communication overhead. It slows things down and adds frustration. But most importantly, it’s nearly impossible to share the complete context. So when one of the two isn’t in the room, the other may have to make crucial decisions without a complete understanding.
Both parties in this arrangement end up equally frustrated: Biologists are beholden to someone else for the final, analytical steps required to interpret the data they’ve worked so hard for. Computational biologists are overworked and often left out of experiment design discussions with massive implications for their ability to deliver.
But at the same time that this situation seems increasingly untenable, there are also reasons to believe it’s only temporary.
One reason is that newly minted scientists are increasingly graduating with a mix of computational and lab skills. End-to-end scientists who can sling pipettes in the morning and python scripts in the afternoon are a growing phenotype that defies the biology/computational biology divide.
But though this small number of scientists grows every year, it’s still a generational shift that will take decades to play out. And in the meantime, because of the consuming nature of each role and the organizational structure of most biotechs, these scientists often have to choose one or the other. So it’s going to take more than just training to make a difference.
A much more rapid change is coming from a different but parallel direction: AI is making computational biology tools more intuitive and accessible to biologists, allowing computational biologists to focus on managing the technical details of the data and developing advanced capabilities. In other words, AI is giving biology back to the biologists.

This reflects an accelerated version of the transformation that earlier standardized primary analysis—turning raw sequences into count matrices and images into quantifiable features—well before the emergence of AI. The most common algorithms have been standardized into building blocks that can be mixed, matched and tweaked by someone who understands the science better than they understand the engineering. Computational biologists or bioinformaticians are still needed to create these building blocks, but running them is becoming increasingly democratized.
Secondary and tertiary analysis were previously resistant to this trend because the building blocks are much more complex and subtle. But this has turned out to be an issue that AI can solve: AI models can arrange the building blocks based on biologists’ intuitive descriptions. And biologists can understand the results well enough to check the AI’s work.
That’s why we designed Sphinx to make this promise a reality. Sphinx allows computational biologists to capture complex algorithms as building blocks and templates, then hand them off to biologists to run. Instead of being the biggest bottleneck to biology, computational biologists who use Sphinx enable their colleagues to work independently and both groups spend their time on what they do best.
Maybe in a few decades every biologist will be as comfortable with code as they are with cell cultures. In the meantime, they can focus on the science while Sphinx takes care of the rest.
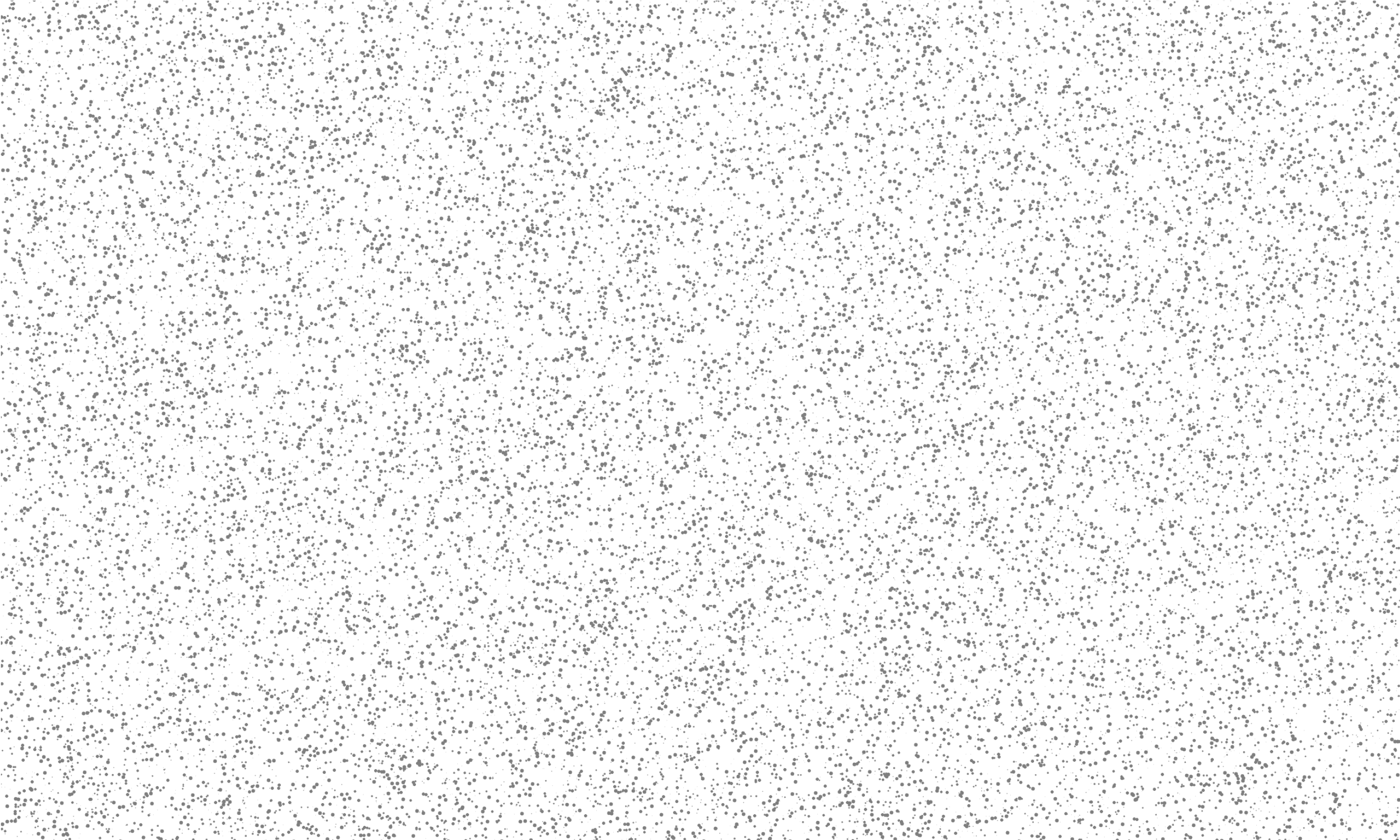
Additional Resources
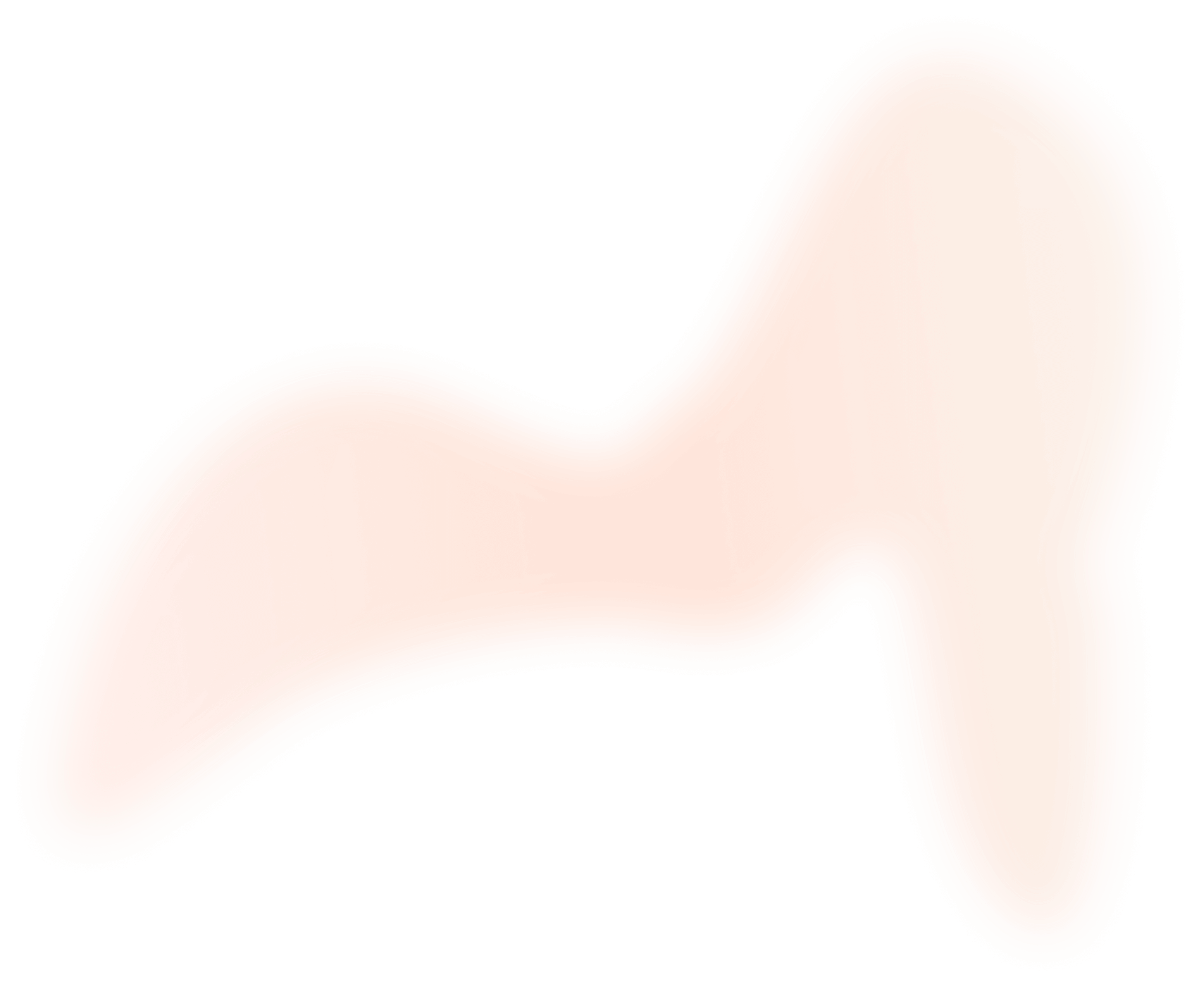